Embodiment of Artificial Intelligence Improves Cognition
February 09, 2012
on
on
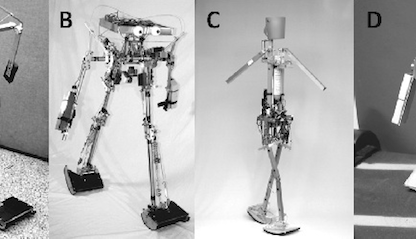
The discussion about the body-mind problem that has kept philosophers busy for over 2 millennia is re-enacted in Artificial Intelligence. Early AI systems were built based on the assumption that intelligence could only be located in the internal control system (mind), now two AI researchers argue that intelligence resides as much in the sensory-motor system (body).
Body-mind problem
Ever since the Greek philosopher Plato there is a dominant school of thought which not only defines humans as dualistic beings but also invariably favors the mind over the body. The latter is considered a dumb vehicle that needs to be controlled by the mind.
It wasn’t until the beginning of the twentieth century that philosophers such as Edmund Husserl and Martin Heidegger argued that cognition resides as much in the body as in the mind. The famous case Heidegger makes is that when I wield a hammer, it isn’t my mind telling my hand what to do. It is my hand, already familiar with the tool, that knows how to work the hammer. The knowledge is located in the body, the mind’s understanding of the act is only a secondary reflection.
Embodiment in AI
The development of AI follows a similar evolution. In classical AI the mechanical system wasn’t given much consideration. Problem-solving was done through tweaking the control system. But now there is an increasing interest in the effects of embodiment on intelligent behavior and cognition.
AI researchers Matej Hoffman and Rolf Pfeifer of the Artificial Intelligence Laboratory at the University of Zurich are early proponents of the importance of embodiment. Recently they published a paper [download link below] in which they argue that embodiment can improve the cognitive functions of AI.
Hoffman and Pfeifer make a compelling argument. They start out with introducing case studies which proof that the mechanical system can perform low-level tasks like locomotion autonomously. They then go on to show that the body can take over or aid in increasingly difficult functions of the control system that can be considered cognitive.
Low-level tasks
The Passive Dynamic Walker [photo above] can walk down an incline without any control or actuation. It relies solely on its mechanical structure and the pull of gravity. Of course, the range of its use is limited but it is an example of a mechanical body that functions through interaction with its environment.
On the other end of the spectrum is Honda’s Asimo robot which relies heavily on its internal control system. It doesn’t take cues from its environment but rather forces its way. Stabilization isn’t accomplished by balanced mechanics but a task of control. Although a far more versatile robot, Asimo requires a lot of computational power and energy.
An alternative to both the limited capabilities of the Walker and the central control paradigm of Asimo is Walknet. This six-legged robot was modeled after insects whose leg movements operate independent of the central nervous system. Walknet’s legs are wired with circuits and sensors. When one leg moves the others get a sign to either bend or stretch. Instead of a central control that has to calculate the position of every leg and send out commands, Walknet’s locomotion depends mostly on an autonomous subsystem and its interaction with the environment.
Embodied categorization
These case studies show that low-level tasks can be off-loaded to the mechanical system. But the body can also aid the control system in its cognitive tasks.
It is difficult to build an AI system that is capable of perceptual categorization, that is, making meaningful distinctions between objects in the environment. Sensory perception is tricky. The qualities of objects change in the eye of the beholder when distance or lightning varies.
In classical AI (or Good-Old-Fashioned AI (GOFAI) as Hoffman and Pfeifer call it) the burden of categorization is on central control. It receives a data stream from the sensors and compares that information to its library of proto-symbols. When the visual sensors record a round object control can categorize it as a circle.
Of course this limits categorization to the proto-symbols stored in the memory bank. Also, control can’t distinct between a large and a small circle because distance isn’t part of the equation.
The researchers cite 2 experiments which show that the more embodied an AI system is, the better it can categorize. When it can interact with its environment through movement and it has different kinds of sensors (touch, visual, audio) it can provide more and better organized sensory data. Making it easier for control to process information.
Towards an integrated system
From the 1950’s onward, philosophers -most notably the French post-structuralists-advocated the notion that body and mind aren’t pure opposites. Instead the lines are blurred to such an extent one can’t properly speak of a dichotomy.
Hoffman and Pfeifer follow a similar train of thought when they say that ‘the distinction between cognitive and sensory-motor starts to blur’.
The researchers propose to understand cognition as the capacity of the cognizer to have thoughts independent of the sensory information it receives about its environment and to contemplate options.
But cognitive processing always starts with the low-level interaction of the body with its environment, they argue. Which is why Hoffman and Pfeifer propose to approach AI as an integrated system rather than a dualistic being.
Hoffman and Pfeifer: The Implications of Embodiment for Behavior and Cognition: Animal and Robotic Case Studies.
Via: technologyreview.com
Photo: Passive Dynamic Walker. Source: The Implications of Embodiment for Behavior and Cognition.
Body-mind problem
Ever since the Greek philosopher Plato there is a dominant school of thought which not only defines humans as dualistic beings but also invariably favors the mind over the body. The latter is considered a dumb vehicle that needs to be controlled by the mind.
It wasn’t until the beginning of the twentieth century that philosophers such as Edmund Husserl and Martin Heidegger argued that cognition resides as much in the body as in the mind. The famous case Heidegger makes is that when I wield a hammer, it isn’t my mind telling my hand what to do. It is my hand, already familiar with the tool, that knows how to work the hammer. The knowledge is located in the body, the mind’s understanding of the act is only a secondary reflection.
Embodiment in AI
The development of AI follows a similar evolution. In classical AI the mechanical system wasn’t given much consideration. Problem-solving was done through tweaking the control system. But now there is an increasing interest in the effects of embodiment on intelligent behavior and cognition.
AI researchers Matej Hoffman and Rolf Pfeifer of the Artificial Intelligence Laboratory at the University of Zurich are early proponents of the importance of embodiment. Recently they published a paper [download link below] in which they argue that embodiment can improve the cognitive functions of AI.
Hoffman and Pfeifer make a compelling argument. They start out with introducing case studies which proof that the mechanical system can perform low-level tasks like locomotion autonomously. They then go on to show that the body can take over or aid in increasingly difficult functions of the control system that can be considered cognitive.
Low-level tasks
The Passive Dynamic Walker [photo above] can walk down an incline without any control or actuation. It relies solely on its mechanical structure and the pull of gravity. Of course, the range of its use is limited but it is an example of a mechanical body that functions through interaction with its environment.
On the other end of the spectrum is Honda’s Asimo robot which relies heavily on its internal control system. It doesn’t take cues from its environment but rather forces its way. Stabilization isn’t accomplished by balanced mechanics but a task of control. Although a far more versatile robot, Asimo requires a lot of computational power and energy.
An alternative to both the limited capabilities of the Walker and the central control paradigm of Asimo is Walknet. This six-legged robot was modeled after insects whose leg movements operate independent of the central nervous system. Walknet’s legs are wired with circuits and sensors. When one leg moves the others get a sign to either bend or stretch. Instead of a central control that has to calculate the position of every leg and send out commands, Walknet’s locomotion depends mostly on an autonomous subsystem and its interaction with the environment.
Embodied categorization
These case studies show that low-level tasks can be off-loaded to the mechanical system. But the body can also aid the control system in its cognitive tasks.
It is difficult to build an AI system that is capable of perceptual categorization, that is, making meaningful distinctions between objects in the environment. Sensory perception is tricky. The qualities of objects change in the eye of the beholder when distance or lightning varies.
In classical AI (or Good-Old-Fashioned AI (GOFAI) as Hoffman and Pfeifer call it) the burden of categorization is on central control. It receives a data stream from the sensors and compares that information to its library of proto-symbols. When the visual sensors record a round object control can categorize it as a circle.
Of course this limits categorization to the proto-symbols stored in the memory bank. Also, control can’t distinct between a large and a small circle because distance isn’t part of the equation.
The researchers cite 2 experiments which show that the more embodied an AI system is, the better it can categorize. When it can interact with its environment through movement and it has different kinds of sensors (touch, visual, audio) it can provide more and better organized sensory data. Making it easier for control to process information.
Towards an integrated system
From the 1950’s onward, philosophers -most notably the French post-structuralists-advocated the notion that body and mind aren’t pure opposites. Instead the lines are blurred to such an extent one can’t properly speak of a dichotomy.
Hoffman and Pfeifer follow a similar train of thought when they say that ‘the distinction between cognitive and sensory-motor starts to blur’.
The researchers propose to understand cognition as the capacity of the cognizer to have thoughts independent of the sensory information it receives about its environment and to contemplate options.
But cognitive processing always starts with the low-level interaction of the body with its environment, they argue. Which is why Hoffman and Pfeifer propose to approach AI as an integrated system rather than a dualistic being.
Hoffman and Pfeifer: The Implications of Embodiment for Behavior and Cognition: Animal and Robotic Case Studies.
Via: technologyreview.com
Photo: Passive Dynamic Walker. Source: The Implications of Embodiment for Behavior and Cognition.
Read full article
Hide full article
Discussion (0 comments)