Does machine learning cause a science crisis?
February 25, 2019
on
on
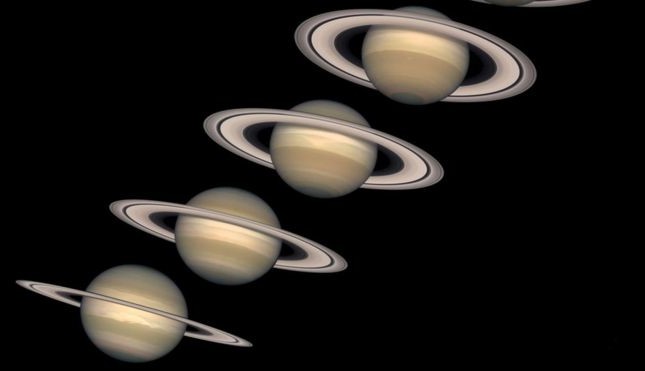
The techniques for machine learning that are used by thousands of scientists to analyse (large amounts of) data, produce results that are misleading and sometimes even completely wrong.
Dr. Genevera Allen from Rice University in Houston (USA) argues that the increased use of such systems contribute to a ‘crisis in science’. She warns researchers that if they do not improve their techniques, they are not only wasting time but also money. Dr. Allen presented her research at the AAAS (American Association for the Advancement of Science) in Washington.
The problem, however, is that the answers that are produced by such research have a significant risk of being inaccurate or even completely wrong, because the software identifies patterns that only exist in that particular dataset and not in the real world.
Often the inaccuracy of such a study is only revealed when the same technique is used on another large dataset and the results don't overlap.
In science there is the growing realisation that there is a reproducibility crisis. According to Dr. Allen this is caused by a large part through the application of techniques for machine learning in scientific research.
This ‘crisis’ refers to the alarming number of scientific results that cannot be reproduced when other researchers carry out the same experiment. The suggestion is that some 85% of all biomedical research that is performed out worldwide, is wasted effort.
One of the causes of the crisis is that algorithms for machine learning have been specifically designed to find ‘interesting’ patterns – with the nearly inevitable consequence that some pattern ot other is discovered, especially when very large datasets are used. Only the question remains whether the pattern actually has a significant meaning – in many cases this is probably not the case.
Source: BBC News
Dr. Genevera Allen from Rice University in Houston (USA) argues that the increased use of such systems contribute to a ‘crisis in science’. She warns researchers that if they do not improve their techniques, they are not only wasting time but also money. Dr. Allen presented her research at the AAAS (American Association for the Advancement of Science) in Washington.
Reproducibility crisis
A growing amount of scientific research uses software for machine learning to analyse the data that has already been collected. This happens across a wide range of research areas, ranging from biomedical research to astronomy. The datasets used for this are very large and very expensive.The problem, however, is that the answers that are produced by such research have a significant risk of being inaccurate or even completely wrong, because the software identifies patterns that only exist in that particular dataset and not in the real world.
Often the inaccuracy of such a study is only revealed when the same technique is used on another large dataset and the results don't overlap.
In science there is the growing realisation that there is a reproducibility crisis. According to Dr. Allen this is caused by a large part through the application of techniques for machine learning in scientific research.
This ‘crisis’ refers to the alarming number of scientific results that cannot be reproduced when other researchers carry out the same experiment. The suggestion is that some 85% of all biomedical research that is performed out worldwide, is wasted effort.
Design
The reproducibility crisis has been growing over the past 20 years, and this is the consequence of the fact that experiments are not thought through well enough to prevent researchers from fooling themselves and only see what they want to see. Broadly speaking, with scientific research, first a (preferably falsifiable) hypothesis is stated, and only then are experimental results examined whether the hypothesis can be confirmed or not.One of the causes of the crisis is that algorithms for machine learning have been specifically designed to find ‘interesting’ patterns – with the nearly inevitable consequence that some pattern ot other is discovered, especially when very large datasets are used. Only the question remains whether the pattern actually has a significant meaning – in many cases this is probably not the case.
Source: BBC News
Read full article
Hide full article
Discussion (0 comments)